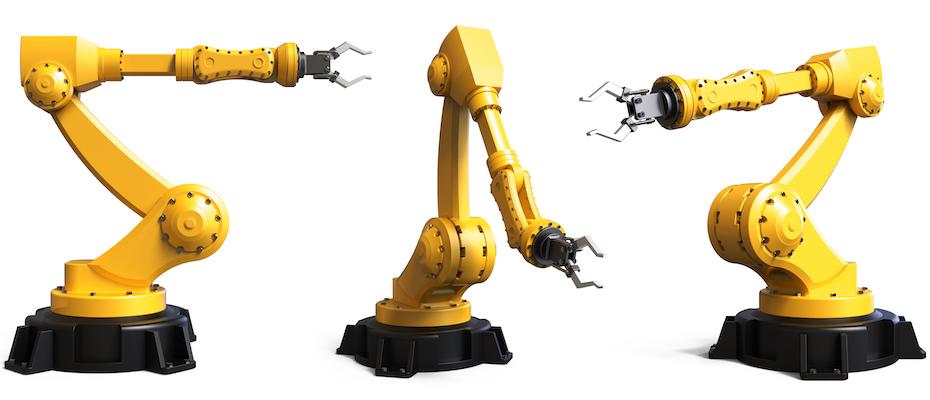
In the battle for e-commerce customers, fortune favors the swift. And with hundreds of billions of dollars at stake and a tight labor market taking a toll on operating budgets, more and more online retailers are looking to automation to speed the order fulfillment process.
Picking is by far the biggest labor cost at fulfillment centers, with the time employees spend walking through a warehouse accounting for more than 50% of total picking time. There are several automation solutions that address the walking time issue, from large automated storage and retrieval systems to our personal favorite: autonomous mobile robots that locate and retrieve bins filled with items and deliver them to a picking station. This type of “goods-to-person” solution is an example of robots performing gross manipulation tasks to bring the bin to a worker, who then selects the individual items needed to fill each order.
One area now generating a lot of excitement in the fulfillment world is the development of robot arms capable of fine manipulation. Robotics researchers consider fine manipulation to be the next big breakthrough, with the dream of a robot that can load a dishwasher without breaking a glass or assemble IKEA furniture seemingly within reach. Fine manipulation utilized in the picking process would, in theory, result in a robot that could correctly pick and pack a wide variety of items in a customer’s order.
And that’s where the hype gives way to reality.
There’s a principle in the field of AI and robotics known as “Moravec’s Paradox.” It refers to the discovery by researchers that, contrary to what you’d expect, high-level reasoning requires relatively little computational power, but low-level, sensorimotor skills require enormous computational resources. In other words, what’s hard for a person—like winning a game of chess—is easy for a machine. And what’s easy for a person—like picking a pen out of a drawer full of pencils—is quite difficult for a machine.
Think about some about the items you can order online: a box of laundry detergent, a jar of peanut butter, and a bag of Halloween candy. You could pick any of these items off a shelf easily with one hand despite the variations in size, shape, and weight. But for a robotic arm, it’s a different story. That box of detergent is a heavy cube, while the peanut butter jar is a breakable cylinder, and the candy is in a flexible sack. Creating an arm with a vision system and a gripper that can identify and pick up each of those items (and thousands of others) correctly every time is a very expensive undertaking—expensive to build, expensive to buy, and expensive to maintain. From a purely economic perspective, they’re just not ready for prime time. People are simply far more efficient and cost-effective when it comes to picking items, hands down.